February 2022: Daniel Blatter
Randomize-then-optimize: nonlinear uncertainty quantification for regularized inversion
Abstract¶
Much of our understanding of the Earth's subsurface comes from physical models produced by inverting geophysical observations made at the surface. Inversion is non-unique and nonlinear, however, meaning there is significant uncertainty in the inverted model parameters. The standard inversion method in geophysics remains regularized inversion despite the fact that it produces single model estimates without a meaningful way to quantify the uncertainty. In this talk I will present 'randomize-then-optimize' (RTO), an uncertainty quantification (UQ) strategy for regularized inversion. This method reinterprets regularized inversion in a Bayesian context, turning these familiar algorithms into Bayesian samplers capable of producing model uncertainty efficiently, even for large geophysical problems. I will discuss the basic theory behind RTO, describe our extension of it to hierarchically sample the regularization strength (which we call RTO-TKO), and show results on field data examples from electromagnetic geophysics.
February 17th, 2022 @ 10:00am PT
Bio¶
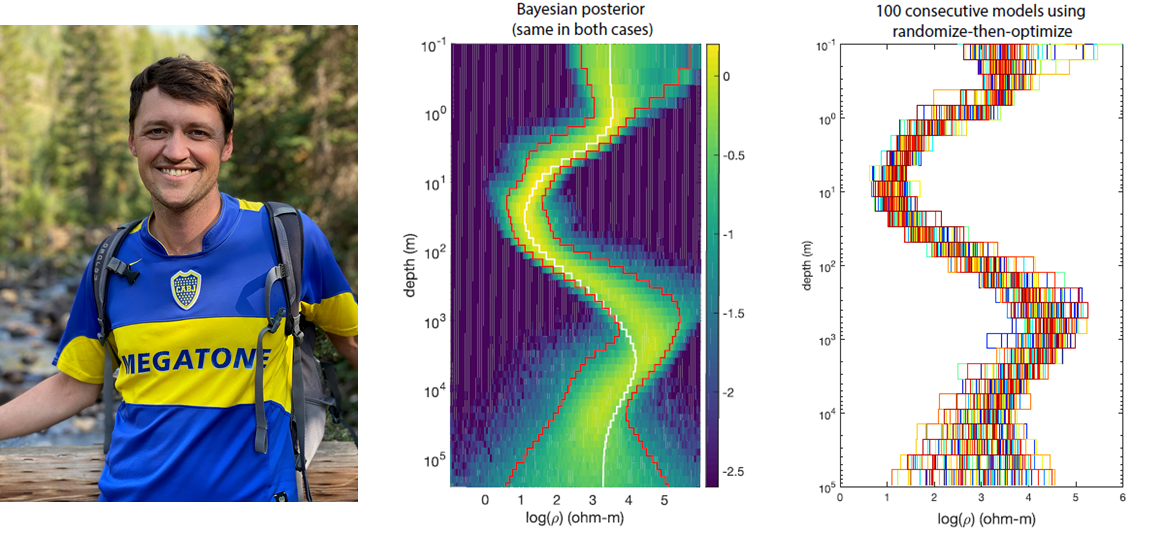
Daniel is the John W Miles postdoctoral scholar in computational and theoretical geophysics at the Scripps Institution of Oceanography, UC San Diego. His research interests are primarily focused in two fields: (1) the lithosphere-asthenosphere system, and the role of fluids in particular, and their relation to plate tectonics; and (2) the development of algorithms capable of quantifying uncertainty in inverted subsurface models inferred from geophysical data. He studied computational mathematics at Stanford University, receiving a Masters in 2015. He did his doctoral studies in geophysics with professor Kerry Key at Columbia University, receiving a PhD in 2020.